Mostrar el registro sencillo del ítem
Exploring semantic deep learning for building reliable and reusable one health knowledge from PubMed systematic reviews and veterinary clinical notes
dc.contributor.author | Arguello-Casteleiro, M. | |
dc.contributor.author | Stevens, R. | |
dc.contributor.author | Des Diz, José Julio | |
dc.contributor.author | Wroe, C. | |
dc.contributor.author | Fernandez-Prieto, M. J. | |
dc.contributor.author | Maroto, N. | |
dc.contributor.author | Maseda-Fernandez, D. | |
dc.contributor.author | Demetriou, G. | |
dc.contributor.author | Peters, S. | |
dc.contributor.author | Noble, P. J. M. | |
dc.contributor.author | Jones, P. H. | |
dc.contributor.author | Dukes-McEwan, J. | |
dc.contributor.author | Radford, A. D. | |
dc.contributor.author | Keane, J. | |
dc.contributor.author | Nenadic, G. | |
dc.date.accessioned | 2022-01-27T10:40:58Z | |
dc.date.available | 2022-01-27T10:40:58Z | |
dc.date.issued | 2019 | |
dc.identifier.issn | 2041-1480 | |
dc.identifier.other | https://www.ncbi.nlm.nih.gov/pmc/articles/PMC6849172/pdf/13326_2019_Article_212.pdf | es |
dc.identifier.uri | http://hdl.handle.net/20.500.11940/15971 | |
dc.description.abstract | BACKGROUND: Deep Learning opens up opportunities for routinely scanning large bodies of biomedical literature and clinical narratives to represent the meaning of biomedical and clinical terms. However, the validation and integration of this knowledge on a scale requires cross checking with ground truths (i.e. evidence-based resources) that are unavailable in an actionable or computable form. In this paper we explore how to turn information about diagnoses, prognoses, therapies and other clinical concepts into computable knowledge using free-text data about human and animal health. We used a Semantic Deep Learning approach that combines the Semantic Web technologies and Deep Learning to acquire and validate knowledge about 11 well-known medical conditions mined from two sets of unstructured free-text data: 300 K PubMed Systematic Review articles (the PMSB dataset) and 2.5 M veterinary clinical notes (the VetCN dataset). For each target condition we obtained 20 related clinical concepts using two deep learning methods applied separately on the two datasets, resulting in 880 term pairs (target term, candidate term). Each concept, represented by an n-gram, is mapped to UMLS using MetaMap; we also developed a bespoke method for mapping short forms (e.g. abbreviations and acronyms). Existing ontologies were used to formally represent associations. We also create ontological modules and illustrate how the extracted knowledge can be queried. The evaluation was performed using the content within BMJ Best Practice. RESULTS: MetaMap achieves an F measure of 88% (precision 85%, recall 91%) when applied directly to the total of 613 unique candidate terms for the 880 term pairs. When the processing of short forms is included, MetaMap achieves an F measure of 94% (precision 92%, recall 96%). Validation of the term pairs with BMJ Best Practice yields precision between 98 and 99%. CONCLUSIONS: The Semantic Deep Learning approach can transform neural embeddings built from unstructured free-text data into reliable and reusable One Health knowledge using ontologies and content from BMJ Best Practice. | en |
dc.language.iso | eng | es |
dc.rights | Atribución 4.0 Internacional | * |
dc.rights.uri | http://creativecommons.org/licenses/by/4.0/ | * |
dc.subject.mesh | Knowledge Bases | * |
dc.subject.mesh | Biological Ontologies | * |
dc.title | Exploring semantic deep learning for building reliable and reusable one health knowledge from PubMed systematic reviews and veterinary clinical notes | en |
dc.type | Artigo | es |
dc.identifier.doi | http://dx.doi.org/10.1186/s13326-019-0212-6 | |
dc.identifier.pmid | 31711540 | |
dc.identifier.sophos | 32758 | |
dc.issue.number | Supplement 1 | es |
dc.journal.title | J Biomed Semantics | es |
dc.organization | Servizo Galego de Saúde::Estrutura de Xestión Integrada (EOXI)::EOXI de Pontevedra e O Salnés - Hospital do Salnés::Oftalmoloxía | es |
dc.page.initial | 22 | es |
dc.rights.accessRights | openAccess | es |
dc.subject.decs | ontologías biológicas | * |
dc.subject.decs | bases del conocimiento | * |
dc.subject.keyword | HP Salnés | es |
dc.typefides | Artículo Original | es |
dc.typesophos | Artículo Original | es |
dc.volume.number | 10 | es |
Ficheros en el ítem
Este ítem aparece en la(s) siguiente(s) colección(ones)
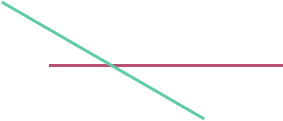