Mostrar el registro sencillo del ítem
Real-time polyp detection model using convolutional neural networks
dc.contributor.author | Nogueira Rodríguez, Alba | |
dc.contributor.author | DOMINGUEZ CARBAJALES, RUBEN | |
dc.contributor.author | Campos-Tato, F. | |
dc.contributor.author | Herrero Rivas, Jesús Miguel | |
dc.contributor.author | Puga Gimenez de Azcárate, Manuel | |
dc.contributor.author | Remedios Espino, David Rafael | |
dc.contributor.author | Rivas Moral, Laura | |
dc.contributor.author | Sánchez Hernández, Eloy | |
dc.contributor.author | Iglesias Gómez, Agueda | |
dc.contributor.author | Cubiella Fernández, Joaquín | |
dc.contributor.author | Fernández Riverola, Florentino | |
dc.contributor.author | Lopez-Fernandez, H. | |
dc.contributor.author | Reboiro Jato, Miguel | |
dc.contributor.author | González Pena, Daniel | |
dc.date.accessioned | 2024-01-02T10:05:07Z | |
dc.date.available | 2024-01-02T10:05:07Z | |
dc.date.issued | 2021 | |
dc.identifier.issn | 0941-0643 | |
dc.identifier.uri | http://hdl.handle.net/20.500.11940/18531 | |
dc.description.abstract | Colorectal cancer is a major health problem, where advances towards computer-aided diagnosis (CAD) systems to assist the endoscopist can be a promising path to improvement. Here, a deep learning model for real-time polyp detection based on a pre-trained YOLOv3 (You Only Look Once) architecture and complemented with a post-processing step based on an object-tracking algorithm to reduce false positives is reported. The base YOLOv3 network was fine-tuned using a dataset composed of 28,576 images labelled with locations of 941 polyps that will be made public soon. In a frame-based evaluation using isolated images containing polyps, a general F-1 score of 0.88 was achieved (recall = 0.87, precision = 0.89), with lower predictive performance in flat polyps, but higher for sessile, and pedunculated morphologies, as well as with the usage of narrow band imaging, whereas polyp size < 5 mm does not seem to have significant impact. In a polyp-based evaluation using polyp and normal mucosa videos, with a positive criterion defined as the presence of at least one 50-frames-length (window size) segment with a ratio of 75% of frames with predicted bounding boxes (frames positivity), 72.61% of sensitivity (95% CI 68.99-75.95) and 83.04% of specificity (95% CI 76.70-87.92) were achieved (Youden = 0.55, diagnostic odds ratio (DOR) = 12.98). When the positive criterion is less stringent (window size = 25, frames positivity = 50%), sensitivity reaches around 90% (sensitivity = 89.91%, 95% CI 87.20-91.94; specificity = 54.97%, 95% CI 47.49-62.24; Youden = 0.45; DOR = 10.76). The object-tracking algorithm has demonstrated a significant improvement in specificity whereas maintaining sensitivity, as well as a marginal impact on computational performance. These results suggest that the model could be effectively integrated into a CAD system. | |
dc.language.iso | en | |
dc.rights | Atribución 4.0 Internacional | |
dc.rights.uri | http://creativecommons.org/licenses/by/4.0/ | |
dc.title | Real-time polyp detection model using convolutional neural networks | |
dc.type | Journal Article | es |
dc.authorsophos | Nogueira-Rodriguez, A.;Dominguez-Carbajales, R.;Campos-Tato, F.;Herrero, J.;Puga, M.;Remedios, D.;Rivas, L.;Sanchez, E.;Iglesias, A.;Cubiella, J.;Fdez-Riverola, F.;Lopez-Fernandez, H.;Reboiro-Jato, M.;Glez-Pena, D. | |
dc.identifier.doi | 10.1007/s00521-021-06496-4 | |
dc.identifier.sophos | 46937 | |
dc.issue.number | 0 | |
dc.journal.title | NEURAL COMPUTING & APPLICATIONS | |
dc.organization | Servizo Galego de Saúde::Áreas Sanitarias (A.S.)::Área Sanitaria de Ourense, Verín e O Barco de Valdeorras - Complexo Hospitalario Universitario de Ourense::Dixestivo||Servizo Galego de Saúde::Áreas Sanitarias (A.S.)::Área Sanitaria de Ourense, Verín e O Barco de Valdeorras - Complexo Hospitalario Universitario de Ourense::Informática | |
dc.relation.publisherversion | https://link.springer.com/content/pdf/10.1007/s00521-021-06496-4.pdf | es |
dc.rights.accessRights | openAccess | |
dc.subject.keyword | CHUO | es |
dc.typefides | Artículo Científico (incluye Original, Original breve, Revisión Sistemática y Meta-análisis) | es |
dc.typesophos | Artículo Original | es |
dc.volume.number | 0 |
Ficheros en el ítem
Este ítem aparece en la(s) siguiente(s) colección(ones)
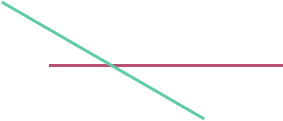